Presented by:
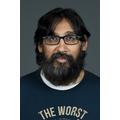
Anson Abraham
No video of the event yet, sorry!
Advances in the technologies and informatics used to generate and process large biological data sets (omics data) are promoting a critical shift in the study of biomedical sciences. While genomics, transcriptomics and proteinomics, coupled with bioinformatics and biostatistics, are gaining momentum, they generate tera- to peta-byte sized data files on a daily basis. These data file sizes, together with differences in nomenclature among these data types, assessed individually with distinct approaches generating monothematic, make the integration of these multi-dimensional omics data into biologically meaningful context challenging. We are in the era of inter-disciplinary data integration strategies to support a better understanding of biological systems and the development of successful precision medicine. Data handling, independent of omics data type, must address issues of data filtering and cleaning (i.e., comparable to data wrangling/cleansing), imputation, transformation, normalization and scaling. Unfortunately, there are no industrial standards in place to unify workflows for any type of omics data. I'll present an overview of some how the ways we're using the PostgreSQL engine, to store the data, process the data in workflows and applying machine learning and analytical aspects when querying the database. The presentation will also provide some of the internals used in postgresql 10 (Azure Database for Postgres), to help scale the data and manage the large datasets and also adhere to HIPAA compliance.
- Date:
- Duration:
- 20 min
- Room:
- Conference:
- Postgres Conference 2020
- Language:
- Track:
- Case Studies
- Difficulty:
- Medium