Presented by:
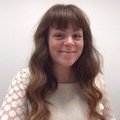
Rebecca Bilbro
Dr. Rebecca Bilbro is a data scientist, Python and Go programmer, teacher, speaker, and author in Washington, DC. With a background in research and a focus on applied machine learning, she specializes mostly in NLP and visual diagnostics for machine learning. An active contributor to the OSS community, Rebecca enjoys collaborating with other developers on inclusive projects like Yellowbrick - a pure Python visualization package for machine learning that extends scikit-learn and Matplotlib to support model selection and diagnostics. In her spare time, you'll find her riding bikes with her family and practicing the ukulele.
No video of the event yet, sorry!
Ironically, one of the most important insights into machine learning in the last decade may have been introduced in a paper not on deep learning or AI, but on databases! In their 2016 SIGMOD paper, Kumar et al. described machine learning as a search for the maximally performing “model selection triple” — i.e. the best combination of features, algorithm, and hyperparameters. “Model selection is iterative and exploratory,” they explain, “because the space is usually infinite, and it is generally impossible for analysts to know a priori which will yield satisfactory accuracy and/or insights.” In other words, doing machine learning in the real world is rarely a linear process, and often fraught with false starts, pivots, and do-overs. So how do you iterate without losing your way?
In this talk we’ll explore techniques that can help make machine learning more informed. It will probably come as no surprise that the first is using a “write-once, read-many” data store (e.g. PostgreSQL, Redis, BTrDB, Mongo), which allows us to experiment with encoding, vectorizing, tokenizing, stemming, and otherwise transforming our data while still preserving its original state. Once we’re confident in our ability to iterate, we then need a suite of tools to help us differentiate our false starts from our successes. And, while it can be tempting to outsource those decisions to proprietary software and black box systems, research and experience alike suggest that human steering allows us to be more strategic in our model selection choices.
This talk features tools from the Python machine learning diagnostics library Scikit-Yellowbrick, which answers the call for open source visual steering tools. For data scientists, Yellowbrick helps evaluate the stability and predictive value of machine learning models and improves the speed of the experimental workflow. For data engineers, Yellowbrick provides visual tools for monitoring model performance in real world applications. For users of models, Yellowbrick provides visual interpretation of the behavior of the model in high dimensional feature space. During the talk we’ll explore how visual diagnostic tools like correlation matrices, radial visualizations, manifold embeddings, validation and learning curves, residuals plots, classification heatmaps, and more can provide visceral cues that enable us to identify better models, faster, and at lower cost to our organizations.
- Date:
- Duration:
- 50 min
- Room:
- Conference:
- Postgres Conference 2020
- Language:
- Track:
- Machine Learning
- Difficulty:
- Medium